What is Google Earth Engine? Google Earth Engine (GEE) is a cloud-based platform that allows…
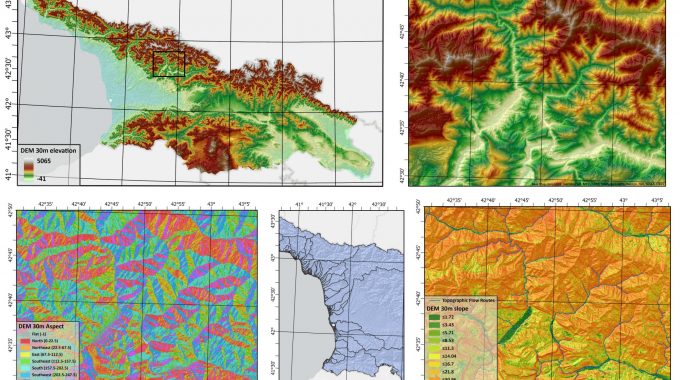
Introduction: Leveraging satellite earth observation data
Remote sensing: The process of detecting and monitoring the physical characteristics of an area by measuring its reflected and emitted radiation at a distance, typically from satellite or aircraft (USGS, 2021).
In short, collecting information from a distance. Through measuring electromagnetic radiation which has interacted with the land, ocean and atmosphere, a wealth of data on the Earth’s system can be gathered to enable data-informed decision making. The term Earth Observation (EO) data is often used interchangeably with remote sensing, both encompassing data obtained from satellites (e.g. Landsat 8, Sentinel-1), airborne-based surveys (e.g. LiDAR) and ground based in-situ measurements. Local ground-based data is often then used to validate (’ground truth’) the airborne-based and satellite datasets.
Remotely sensed data is readily available and typically open source for many metrics online including topography, climate, population, forest cover, soil characteristics and carbon. At Síor we utilise such big data to aid our geospatial analysis through the use of our IT infrastructure. Using modelling and geoprocessing tools, remotely sensed data derivatives can be generated, providing datasets otherwise complex to collect first-hand.
A key remotely sensed satellite data set utilised daily at Síor is the Shuttle Radar Topography Mission (SRTM) Digital Elevation Model (DEM). Although with a 30m spatial resolution, it is not suitable for all projects, this data set can be analysed using our GIS software to produce a range of derivatives, examples as seen below.

I recently ran such a topographical analysis on ArcPro for the Congo River basin, the second largest basin in the world, for my MSc thesis.
Access to geospatial data from satellites has grown hugely in the last few decades. The potential can be vast, especially if combined with machine learning techniques and approach. Often in remote sensing, the desired value is not what the raw data measured; the use of computational methods and algorithms (e.g., machine learning methods) are required to produce an operational dataset.
At Síor, we utilise a range of remote sensing datasets every day for a variety of applications across our multiple disciplines and are constantly expanding our knowledge and use of earth observation data.
Comments (0)