What is Google Earth Engine? Google Earth Engine (GEE) is a cloud-based platform that allows…
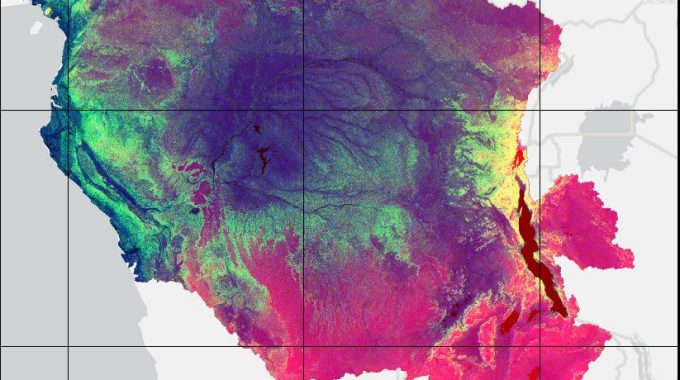
Introduction: Machine learning and environmental science: an emerging field
You will undoubtedly have had more exposure to machine learning than perhaps you realised; from Alexa and Siri, journey planning and fraud detection. Machine learning is fast becoming a major part of modern day living, contributing to the ease of everyday tasks. However, it is also increasingly being used to assist with realms of environmental science.

Machine Learning: In essence, machine learning refers to training computers to recognize patterns in data (e.g., through classifying and clustering), to then make predictions. The computer leverages the patterns learned during training through the use of algorithms (i.e., a set of rules derived by the computers to solve calculations or problems). These algorithms can then be combined to form complex machine learning models, which result in a mathematical representation capable of representing a real-world process.
Two main forms of machine learning are:
- Unsupervised learning: Approaching problems with little knowledge of what the output should look like, deriving structure from the data where the effect of the variables is not known. e.g., clustering. Online shopping websites, like Amazon, use unsupervised learning in the form of clustering to tailor the site to the user preferences.
- Supervised learning: There is a relationship between inputs and outputs, therefore given a dataset it is known what the current output looks like.
- Regression: variable to predict is numerical e.g., linear regression model. For example prediction of future e.g. temperature.
- Classification: variable to predict is part of a number of pre-defined categories e.g., decision tree-based classification . A real-life example would be computers classifying an email as spam.

Although machine learning has been around since the 1950s, it is rapidly advancing today due to the vast availability of big data, processing power, software, improved algorithms, and an increasingly connected globe. These advancements have resulted in machine learning becoming more intertwined in our everyday lives. The increasing availability of big data has enabled the successful deployment of machine learning to solve complex tasks and has contributed to the establishment of really effective machine learning platforms, which in turn streamline the process of building, training, validating and comparing the performance of different machine learning models.
The increasing supply of earth monitoring (big) data, which is available through remote sensing, has also played a big role in increasing the potential for machine learning to be applied to complex, sometimes untapped, environmental problems.
Machine learning and environmental science: an emerging field:
Machine learning and artificial intelligence (AI) can, therefore, “sense their environment, think, learn, and act in response to what they sense and their programmed objectives”. (Harnessing Artificial Intelligence for the Earth. World Economic Forum)
Machine learning can now predict patterns more efficiently than humans, providing a great potential opportunity with environmental policies, including those surrounding climate change. In particular, machine learning can be used as an additional set of modelling tools to predict environmental threats and hazards. Examples include modelling conditions of ice sheet melt, monitoring endangered species non-invasively and identifying landcover change and deforestation.
“Essentially all models are wrong but some are useful“
George E P Box
Models are essentially a simplification of reality and can vary in their complexity. However, they can aid understanding of the earth system through prediction and explanation of various components. In this regard, machine learning is starting to become the new paradigm for an increasing number of environmental challenges.
Here at Síor, we are exploring how machine learning can be used to aid environmental risk evaluation, utilising both spatial and linear data in this emerging field.

Comments (0)